The Coin Toss and the Love Triangle
There are two flavors of uncertainty in our lives. Math helps with both.
By Simon DeDeo
I returned, and saw under the sun, that the race is not to the swift, nor the battle to the strong, neither yet bread to the wise, nor yet riches to men of understanding, nor yet favour to men of skill; but time and chance happeneth to them all.
—Ecclesiastes 9:11, King James Bible (Pure Cambridge Authorized Version)
“Chance”appears to name a single, unitary thing. But its genealogy, its family history, turns out to be a tangled one. One way to understand its branching origins is to turn to literature: We may look, in turn, to two very different novels.
Anton Chigurh, the antagonist of Cormac McCarthy’s novel No Country for Old Men, forces his victims to guess the outcome of a coin toss, taking their life if they guess in error. McCarthy’s villain forces blind chance into his victims’ lives in the most brutal way. That chance is entirely contained, not in Chigurh, but in the toss — in nature itself. This is one source of uncertainty.
To understand the second source, we travel as far as possible from McCarthy’s American Southwest. The first volume of Henry James’s The Wings of the Dove ends with Milly Theale, a wealthy American heiress, visiting the National Gallery in London. To her surprise she sees an acquaintance, Merton Densher, in the company of her best friend, Kate Croy. The plot of the book from this point forward hinges on a single question: will Milly learn what the reader already knows — that Merton and Kate are in love and secretly engaged to be married.
In the sequence, told from Milly’s point of view, we see how Kate — caught sharing an intimate afternoon — acts in such a way as to generate an alternate hypothesis for her friend: that Merton may well be keen on her, but that she, Kate, is not keen on him.
Here are uncertainties not otherwise found in nature: probabilities about probabilities, beliefs about beliefs held by others.
For many of us, the material world of the coin toss may be a byword for chance. But the cognitive world of the love triangle is just as fraught and, at its limits, just as much a form of chance as a tumbling coin. We humans are capable of introducing a degree of uncertainty both dizzying and unavoidable.
Therefore, Ecclesiastes was right twice over. Uncertainties — both natural and human — must be dealt with even by the swift, strong, wise, and able. Our objective is to demonstrate the depths of each kind of uncertainty and to introduce mathematics that can help us come to terms with both.
I. The Coin Toss
What’s the most you ever saw lost on a coin toss?
— No Country for Old Men, Cormac McCarthy (2005)
Sufficiently many fictional murderers have toyed with their victims that, today, the idea borders on cliché and parody. But Chigurh’s game chills us still, perhaps because of the concentrated form of his message. His impartial, deadly coin toss is a reminder of the dominance of chance in our own lives.
From the accidents we have avoided or been met with, to the relationships we have formed and the institutions we have come to be associated with — each fact about ourselves appears to depend on a series of events, any one of which could have gone another way. To stand on top of a tower of such coincidences and look down gives us a hint of vertigo. Which aspects of life are real, we ask, and which simply luck?
This question is, on the one hand, at the heart of much literature and art; on the other, it is a hardheaded question in the mathematical sciences.
Many fictional murderers have toyed with their victims.
In particular, the branch of mathematics known as information theory concerns itself with how to describe chance and uncertainty. It reconciles the unlikeliness of any particular life with the intuitive sense that the shape of one’s life is not simply a matter of chance.
To see how, let us begin at the beginning, at the base of the tower of coincidences. Suppose Chigurh’s coin toss is fair — equally likely to come out heads or tails. What are the possible strategies his victim should consider? Always guess tails? Heads? Some alternation?
Should the toss be fair, no strategy is better than another. Indeed, strategies are indistinguishable from each other. This is due to the symmetry of the problem: We can swap the labels on each side of Chigurh’s coin at any time and thereby convert any prescription into any other. In McCarthy’s desert landscape, strategy and reason are meaningless. The vertigo of chance is upon us.
This, of course, doesn’t describe the world we normally confront. In our world there is predictability, with a preference between outcomes¹ and the possibility of rational choice. Broken symmetries make reason relevant to our lives.
But this doesn’t rescue us from the vertigo of chance. Suppose, for example, Chigurh’s coin is slightly more likely to come up heads. Now, strategies are distinguishable. For a heads-biased coin, the preferred and most rational strategy is always to guess heads.
In the course of your life you need to make many decisions. What if you had to guess the coin toss more than once? Consider tossing the biased coin a thousand times. Since each toss is independent of the one before, the most likely outcome is the repeated occurrence of the most likely outcome of each one separately. And thus, of all the possible histories we could foresee, the strangest sequence of all, an unbroken run of heads:
HHHHH … H, 1,000 times
is the single most likely. Our intuition is that such a sequence will never, in fact, occur.
And of course we are correct. Fix the bias of the coin at 60 percent heads. The chance of heads on a first toss is (by definition) 6 in 10; of two heads in a row, a little over 1 in 3. The chance of three heads in a row is only a little better than 1 in 5.
In McCarthy’s desert landscape, strategy and reason are meaningless.
The chances of an unbroken run decrease exponentially; the chance of ten heads in a row is less than 1 percent, and a few more doublings suffice to place the chances beyond the astronomical. It is unlikely to see an unbroken run of heads in 80 tosses, even if one completes such a sequence once every second for the 13 billion years the universe has existed.
We’ve established that a string of unbroken heads is extremely unlikely. But any other history is even more so. As time passes, every narrative becomes an extreme rarity. Despite the existence of a most rational choice, the particulars of your life describe a very unlikely path. The biased coin, which signifies the possibility of reason, does not relieve our vertigo after all.
Yet we also know that some things are routine, expected — even, at times, part of our birthright — and others less ordinary. A friendship might depend on having shared a freshman seminar, but is it so unlikely to have made a friend in college?
This intuition has a mathematical grounding in what is called the typical set. The typical set is the mathematics behind our feeling of normalcy. It reconciles reason and chance by linking the nature of the singular event to the properties of the history it belongs to. More than biased probabilities, it challenges the vertigo of pure chance.
Consider the space of all possible histories: an exhaustive list of every sequence of events that might have occurred. Every coin toss, every decision ever made. The typical set bounds a very small region in this space and describes the path that we, as time goes on, are increasingly likely to follow. Given a prescription for the probabilities of individual decisions, the typical set picks a list of histories whose rates of uncertainty accumulation become increasingly close to each other, and match, on the average, the intrinsic rate of the probabilities themselves².
Let’s return to the freshman seminar. Your college life is a series of chance events whose probabilities are set by a finite list of constraints: your major, your age, and so on. As your college days run on, the set of possible histories you are likely to experience converges with those found in the typical set your constraints define. Each individual history is rich in idiosyncrasy while being drawn from a narrowly circumscribed set of possibilities that is much smaller than the space of all that might happen³.
Despite the existence of a most rational choice, the particulars of your life describe a very unlikely path.
We are left in a profoundly ambiguous place. The typical set rescues normalcy but also dictates typical lives, common stories: boy meets girl, dog bites man. This wisdom was also known to the author of Ecclesiastes, who wrote that there was “nothing new under the sun.” To the swiftest, the race might go once, or even twice, but on the longest scales of time no streak is left unbroken.
At the same time, our pasts and our futures — even our most likely futures — are, in their details, profoundly unlikely things.
An information theorist may not know the exact world we live in, but she does know that, in the long run, it’s a world in the typical set. And she also knows that she doesn’t know anything else⁴.
So much for coin tosses and freshman seminar assignments: things external to ourselves, driven by the chances of the physical world⁵. These material things, however, are not the only — or even the most important — sources of life’s uncertainty. To see that, we turn from Cormac McCarthy’s American Southwest to Henry James’s London.
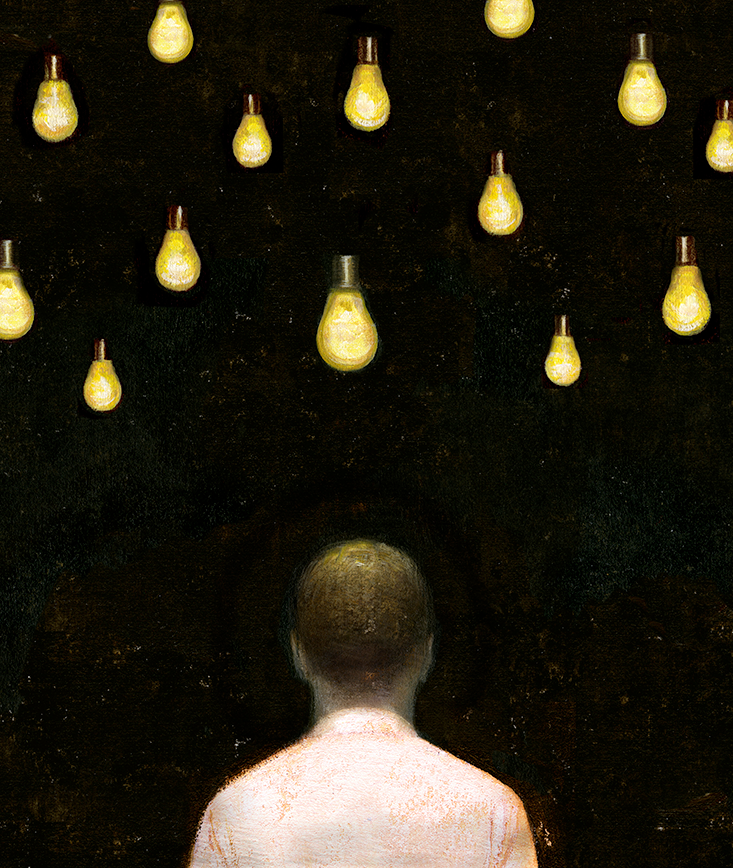
II. The Love Triangle
Little by little indeed, under the vividness of Kate’s behaviour, the probabilities fell back into their order.
— The Wings of the Dove, Henry James (1909)
It is a remarkable fact about the structure of our reasoning that probabilities can be understood to represent not only chances of outcomes in the physical world, but the strength of the convictions we hold within our minds⁶. This has been known explicitly only since the 1940s, when the physicist Richard Cox derived the laws of probability from a set of logical postulates. His work was then adapted by key figures such as Edwin Jaynes (another physicist) to found an account of chance based on degrees of belief — to go from biased coins to biased thoughts about them.
It is under this latter understanding of chance that James’s narrative best shines. Milly must make sense of the unexpected: Merton and Kate together in the National Gallery. Under the influence of Kate’s posturing, she must form a set of beliefs — a set of “probabilities,” concerning beliefs about beliefs. James’s self-reflexive prose abounds with difficult-to-track higher-order thoughts — perhaps this is why it was once said of James that he chewed more than he bit off.
Our pasts and our futures are, in their details, profoundly unlikely things.
Although remote from the raw chance of the physical world, mathematics can also help us understand how to describe these beliefs and show us how they can start to resemble raw chance.
To begin with some notation, let us represent the fact that person X believes sentence s in the form BX (s), which is read as “X believes that s”⁷.
The recognition that takes place between Milly and Merton and sets the narrative in motion can be described this way. Merton sees Milly first; he is of course aware of this, and so we can write:
BMerton(“Merton sees Milly”)
Or, in words, “Merton believes that Merton sees Milly” (it will save us a great deal of trouble if we avoid pronouns for a spell).
Milly then sees Merton. As she is also self-aware,
BMilly(“Milly sees Merton”)
When, as the narrative tells us, she can see that Merton can see her, we have
BMilly(BMerton(“Merton sees Milly”))
Or that “Milly believes that Merton believes that Merton sees Milly.” Not only is this recognition mutual, but so is the recognition of this recognition, so that
BMilly(BMerton(“Milly sees Merton”))
And, indeed, this recognition itself is also known to Merton, so that
BMerton(BMilly(BMerton(“Milly sees Merton”)))
Aided by the mathematical notation, one can see that these towers are potentially infinite in extent, with true and false beliefs possible at each point.
Game theorists — in a beautiful simplification — use the term common knowledge to refer to cases where sentence s is true, and any arbitrarily nested belief functions referring to the state of knowledge of individuals concerning s are also true. Common knowledge is often associated with social norms such as obeying traffic lights and being polite, but here we can see the infinite tower emerge, at once, in the meeting of gazes.
Not all forms of belief are commonly held; indeed, it is the failure of common knowledge that drives many narratives (including those of Othello, Hamlet, and nearly all of Shakespeare’s comedies).
It is only after Milly and Merton have recognized each other, in other words, that the games begin. After the awkward encounter, Milly invites both Merton and Kate to her hotel so as to appear to take Kate’s hint that the encounter must seem to Milly “queer,” but that it has a simple interpretation. Milly does this in part because she believes the best solution is to adopt and emphasize her “native wood-note,” her own spontaneous, American attitudes. This, she feels, will ease the strain of her stumbling on a scene of unrequited (that is, Merton’s) love.
In short: Milly believes that Kate believes the scene has induced a belief in Milly; Milly, holding beliefs about her own beliefs, attempts to induce a new belief in Kate. Such patterns of thought are real, if delicate; they are difficult to represent properly in English (though of course James tries) but more than amenable to the formalism above.
Milly reasons that, becauseBMilly(BKate(BMilly(“something’s queer”)))```
and
BMilly(BMilly(“one should be straight-forward”) is the best response),
the awkward predicament can be resolved by acting such that
BKate(BMilly(“with time, an innocent explanation will emerge”)).
Milly is proud of the complexity of her reasoning⁸, motivated by a noble desire to be a good friend to Kate. She is unaware, of course, that the English girl, nesting all of Milly’s beliefs one level deeper and responding appropriately, is able to conceal the essential truth: that Merton’s love is in fact requited⁹. At each stage, these belief functions carry with them probabilities — degrees of belief — that multiply together in increasingly complex ways.
The typical set was the star of Section I: It gave us a handle on the nature of uncertainty. The sources of that uncertainty were drawn from the material world. Here in Section II we have seen how to describe the emergence of uncertainty among thinking, competing agents. The extension of chance to the social world requires a new set of mathematical tools. These tools show us plainly the potential for spiraling complexity, for reflexivity, which we can now name precisely: BMilly(BKate(BMilly…
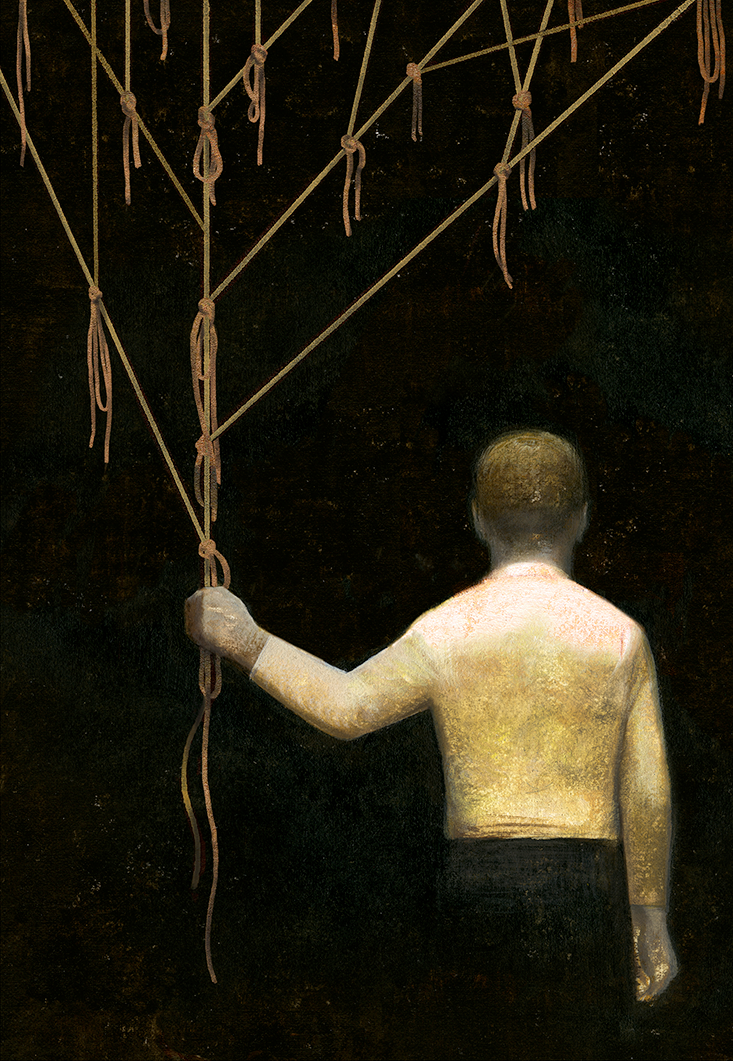
III. The Limits of Reflexivity
At sufficient complexity, social and natural uncertainty, both in principle and in practice, become indistinguishable. While readers might find Wings of the Dove difficult (if rewarding), they are not alone if forced to rely on the Merchant-Ivory version of James’s subsequent, and even more oblique novel, The Golden Bowl.
Put another way, our cognition, so limitless in the development of the natural sciences, seems the most fallible when, in walking down the street, we meet a stranger coming from the other direction, and, symmetrically polite, both move left and right in a frustrated attempt to make way.
The extension of chance to the social world requires a new set of mathematical tools.
It occurs to us that the best solution can often be the leaving of things to chance. When our social environment demands we take into account too many reciprocal beliefs about beliefs, we become — against our better knowledge — mystics. We attribute chance and randomness to others even as we fail to attribute it to ourselves. The minds of others become too complex, and we treat the social world as we do the natural one — as fundamentally unknowable, fundamentally subject to chance.
Navigating the limits of our ability to judge the degree of certainty in our own beliefs and those of others is a defining human characteristic. With this understanding, the point at which one crosses from theories of mind to theories of chance is a balance of innate ability and a philosophical choice about what it means to lead a good life.
One might imagine a science fiction device — a probability meter — that would measure the differential contribution of nature and humankind to the uncertainty of an outcome. How much uncertainty in the average corporate boardroom is due to nature (e.g., the chances of bad weather delaying a shipment of parts) and how much to the strategic creation of uncertainties by human participants (e.g., the refusal to disclose final production targets to one’s suppliers)? Of course, such a meter could wreak havoc in any real boardroom — and itself be a source of its own readings.
Social and natural uncertainty are like natural and anthropogenic sources of carbon dioxide. When CO2 reaches the atmosphere, its source makes little difference. But there are essential differences in our ability to manage social and natural uncertainty. Our modern world of technology and planning has transformed many natural uncertainties into near-certainties. The same, however, cannot be said of higher-level aspects of human interaction.
Not that we aren’t trying. Today, machines manage much of our response to chance. They do so often by a low-level approximation of our own ideals of reasoning. Modern algorithms respond not only to inputs from their environment but also to degrees of belief in those inputs.
Machine learning systems that use this so-called Bayesian approach not only monitor the antilock brakes on modern cars and fly-by-wire passenger jets, but have spread far into the social domain. Among other things, they provide estimates of (and degrees of belief in) a student’s scholastic abilities (as part of automated essay-grading algorithms used by the Educational Testing Service for the SAT exam) and the romantic compatibilities of couples (as part of the algorithms developed by the online dating service eHarmony).
Computers, however, have shown far less ability than humans in the modeling of higher-order systems. While they can estimate with high accuracy the optimal move in a game of chess, they have performed poorly in games such as poker that require estimation not only of the facts at hand but of the beliefs of other players concerning those facts.
Modern algorithms respond not only to inputs from their environment but also to degrees of belief in those inputs.
Machines that play a naively “optimal” poker — folding with poor hands and persisting with better ones — can beat a simpleminded human player by better estimation of the odds. But they lose disastrously to a more expert player who can learn the underlying strategy and, by reference to the machine’s tolerance for risk, bluff more precisely.
Since we have allocated to machines reasoning tasks that far exceed our own abilities, since each successive limit to the power of computers relative to those of human reason has fallen, we might well ask: Can we expect this last domain in which properly thoughtful humans remain the unchallenged experts to remain inviolate? Will machines eventually diagram The Golden Bowl for us? And perhaps — let us speculate — produce new solutions to the dilemmas faced by its characters?
More lucrative avenues than literary criticism suggest themselves. Will corporations someday put supercomputers into boardrooms as well as their research labs to better anticipate and maneuver against their competition?
Though few have gotten rich betting against technological progress, this final step to fully automated reflexive knowledge of other minds seems far off. There are even reasons to believe that the search would amount to the construction of a conscious being or would pose questions fundamentally unanswerable to man and machine alike¹⁰. Should such a thing occur, our world would become, in an instant, unrecognizable. But the words of Ecclesiastes would still remain: Time and chance shall happen to them all — machine and creature alike.
Footnotes
- The inscriptions on the two sides of a coin have different forms and weights; this difference imprints itself on how coins behave in the real world, as can be seen by balancing a dozen pennies on their edge, and thumping the table to make them fall. A tossed coin is less sensitive to these effects, but (more seriously) is sensitive to the side facing upward at the beginning of the toss — the bias is roughly 51–49 in favor of the coin showing the side that faced up at the start.
- The relationship between a history in the typical set and the underlying chance events that produce it is somewhat akin to the train conductor who makes up time along his route. In the short run, one might be delayed — or arrive ahead of schedule; but on the longest journeys, one finds the train pulls in exactly as expected.
- How to best adapt the mathematics of probability to the cognitive and social worlds remains a challenging problem and places us at one of the major research frontiers. Two assumptions in particular, technically known as stationarity and ergodicity, play central roles here. My colleague Ole Peters, at the London Mathematical Laboratory, has been at the forefront of asking what we might need to do if these simplifying assumptions fail, in part by careful study of the St. Petersburg paradox, an apparent failure of human reasoning to abide by mathematical principles.
- Leibniz introduced us to the idea of possible worlds and was memorably parodied as Dr. Pangloss in Voltaire’s Candide, who teaches his student that we live “in the best of all possible worlds.”
- The “chance” in the King James Version translation is the noun, ôÌÆâÇò (pega) in the original Hebrew, etymologically derived from the word for “impact.”
- It is not difficult to describe games where the mathematically rational choice is at odds with the sensible one we would make ourselves. One example is the Allais paradox. Question one: Which would you prefer? A million dollars guaranteed or an 89 percent chance of $1 million and a 10 percent chance of $5 million? (And thus a 1 percent chance of nothing.) Question two: Would you prefer an 11 percent chance of $1 million or a 10 percent chance of $5 million? If you prefer the first option for question one and the second option for question two, your choice-making violates some of the basic assumptions that underlie the mathematization of reason presented here.
- Here we adopt the semantic approach of the Interactive Epistemology notation given by Aumann, Int. J. Game Theory (1999) 28:263.
- We assume, as James does in this passage, that Milly can alter her own beliefs at will. Not a trivial assumption: A common human predicament is the desire to change a detrimental belief. Such desires are familiar in the religious realms — the struggle for faith — as well as the social ones, where self-help books encourage one to practice staying positive (i.e., holding certain beliefs about the future) against one’s prior, learned inclinations. Such struggles are not uncommon in science, either: Scientists often report a frustrated desire to reconcile their “naive” beliefs with their scientific ones.
- Merton Densher has very little idea what is going on and spends most of his time in the nested belief functions of the two ladies. Whether this represents an amusing comment on the powers of Edwardian-era male reasoning or a failure of imagination on the part of Henry James is left as an exercise for the reader.
- A machine capable of reasoning about reasoning — capable of forming beliefs about its beliefs, as is necessary for the kind of higher-order reasoning seen in the writing of Henry James — also becomes subject to one of the most mysterious mathematical results of the 20th century: the so-called Incompleteness Theorems, first explicitly formulated by Kurt Gödel in 1931. One can see this informally in the self-referentiality that the BX(s) formalism allows and can imagine sentences that refer to, for example, the believability of sentences that deny their believability; more rigorously, one can note the possibility of modeling a primitive arithmetic with the syntactic rules available.
Simon DeDeo is a Research Fellow at the Santa Fe Institute. His work, on the cognitive structure of natural and artificial systems, is supported by the National Science Foundation and the Emergent Institutions Project